Have you ever wondered why Booking.com matches your preferences so well? Or why can TripAdvisor suggest a perfect restaurant choice? It’s all thanks to advanced recommendation systems that analyze user behavior, past decisions, and travel trends.
In this article, we’ll look at using different recommendation systems to personalize offers and increase customer satisfaction. We’ll show examples of successful implementations and suggest the best places to position them on your website.
What are travel recommendation systems?
Recommendation systems in travel are advanced tools that use artificial intelligence algorithms to analyze data about preferences, previous choices, and user behaviors to present personalized suggestions for accommodations, tourist attractions, or restaurants. Thanks to data analysis, they function like virtual travel advisors who know the customer’s taste and can suggest exactly what they’re looking for, often before they can formulate it themselves.
When many of us feel overwhelmed by the mass of information and available options, recommendation algorithms filter offers and display the most relevant ones, saving our time and increasing our satisfaction with our choices.
Popular Travel Recommendation Types
In our previous article, “Product and Service Recommendation Systems: Key Benefits and Real-World Applications,” we discussed the most popular types of recommendation systems. Today, we’ll examine how these mechanisms are applied in the tourism industry, offering travelers personalized experiences
1. Popularity-Based Recommendation Systems
Popularity-based recommendations are a proven way to increase conversion in the tourism industry. The system shows users the places and destinations most frequently chosen by other customers. These can include the most frequently booked hotels (popularity-based ranking), highest-rated attractions (ratings-based approach), destinations gaining the most interest in a given season (seasonal forecasting techniques), or the popularity of places in a specific city (location-based recommendations).
While popularity in e-commerce is often measured by the number of products sold or customer ratings, in tourism, this mechanism also takes into account seasonality and context. Popular winter destinations differ from summer hits, and popular business hotels differ from family resorts.
2. Cross-Selling Recommendations
This method suggests additional services complementing the customer’s main reservation, thereby increasing the order value. These contextual session-based recommendations use information about the user’s current behavior to recommend appropriate services and products.
In traditional e-commerce, cross-selling involves offering accessories for a purchased product (for example, a case or headphones for a smartphone). In tourism, this mechanism is more complex and contextual. The system analyzes the travel destination, nature, and selected accommodation specifics to suggest relevant add-ons.
Some examples of how this works in the tourism industry:
- Offering airport transfers after a customer books a hotel
- Suggesting travel insurance that matches the type of trip
- Offering car rentals at the destination
This approach works well at different stages of the customer journey:
- While browsing offers – “Complete your stay with…”
- During the booking process – “Add to your booking…”
- After purchase – “Personalize your trip with…”
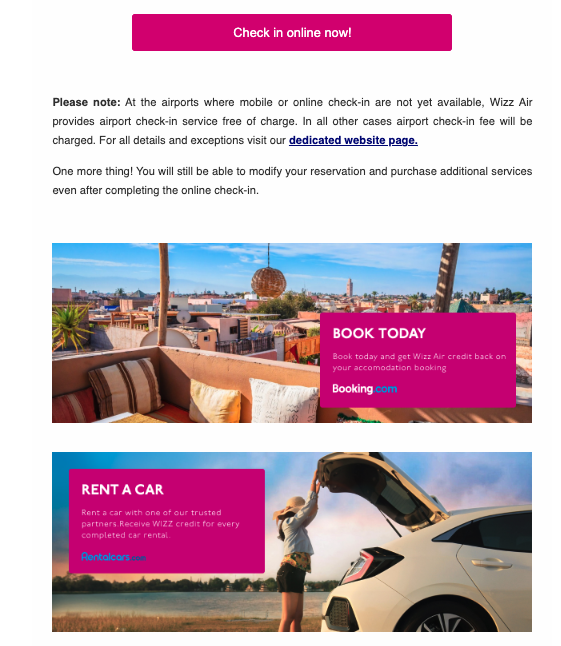
3. Behavior-Based Recommendations
Recommendation systems that use customer data are among the most powerful personalization tools in the tourism industry. The system analyzes the behavior patterns of users with similar preferences, creating recommendations like “Customers who booked this hotel also visited…” Instead of relying on predetermined rules, the algorithm learns user behavior patterns based on actions such as visiting hotel pages or browsing categories.
The system can recommend accommodations based on users’ previous purchase histories. This is particularly effective for building long-term relationships with customers and people who travel regularly, such as for annual vacations or frequent business trips.
Platforms like Booking.com successfully use this method, analyzing both group behaviors (“tourists similar to you chose…”) and the customer’s individual purchase history (e.g., automatically promoting wooden cabins for a user who regularly chooses this type of accommodation).
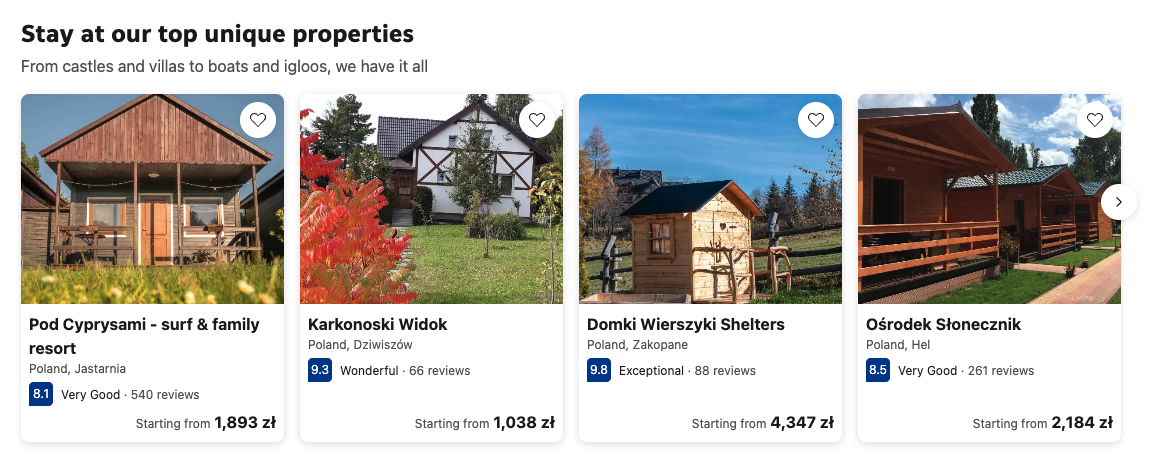
This combination works like an experienced travel agent who knows their clients’ preferences and can suggest new, relevant options based on the experiences of similar travelers.
4. New Offerings Recommendations
Recommendations for new offerings in the tourism industry play a somewhat different role than in traditional e-commerce. While new products appear regularly in online stores, in tourism, a “new offering” might be a newly opened hotel, a recently introduced flight route, or a completely new type of experience. In this case, algorithms rely on features of the offer and similarity methods.
Such appropriately personalized recommendations of new offerings can effectively inspire another trip or the discovery of an unknown destination. This is also an ideal suggestion for travelers seeking new, unique adventures and experiences.
The key, however, is matching – a new luxury hotel won’t interest a customer traveling on a low budget, but it might be attractive to someone who regularly chooses premium accommodations.
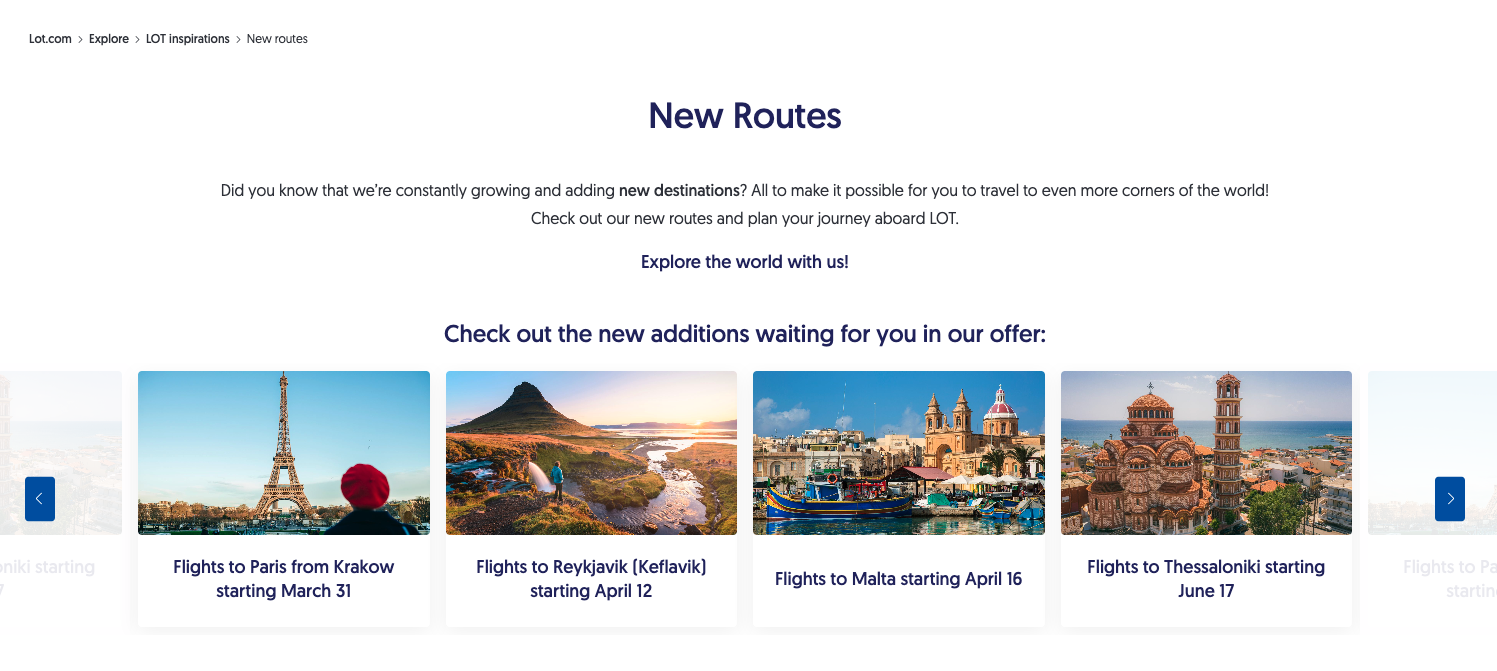
5. Location-Based Recommendations
Recommendations using geolocation data can become a key element of the entire travel planning and execution process. The system can use location- and clustering-based recommendations, which group places based on geographic features, creating “routes” or “packages” of tourist experiences.
This type of recommendation in tourism takes various forms, such as:
- Suggesting attractions near a booked hotel
- Showing restaurants in the vicinity of the traveler’s current location
- Recommending alternative accommodations in a preferred neighborhood
- Informing about events taking place during the planned stay
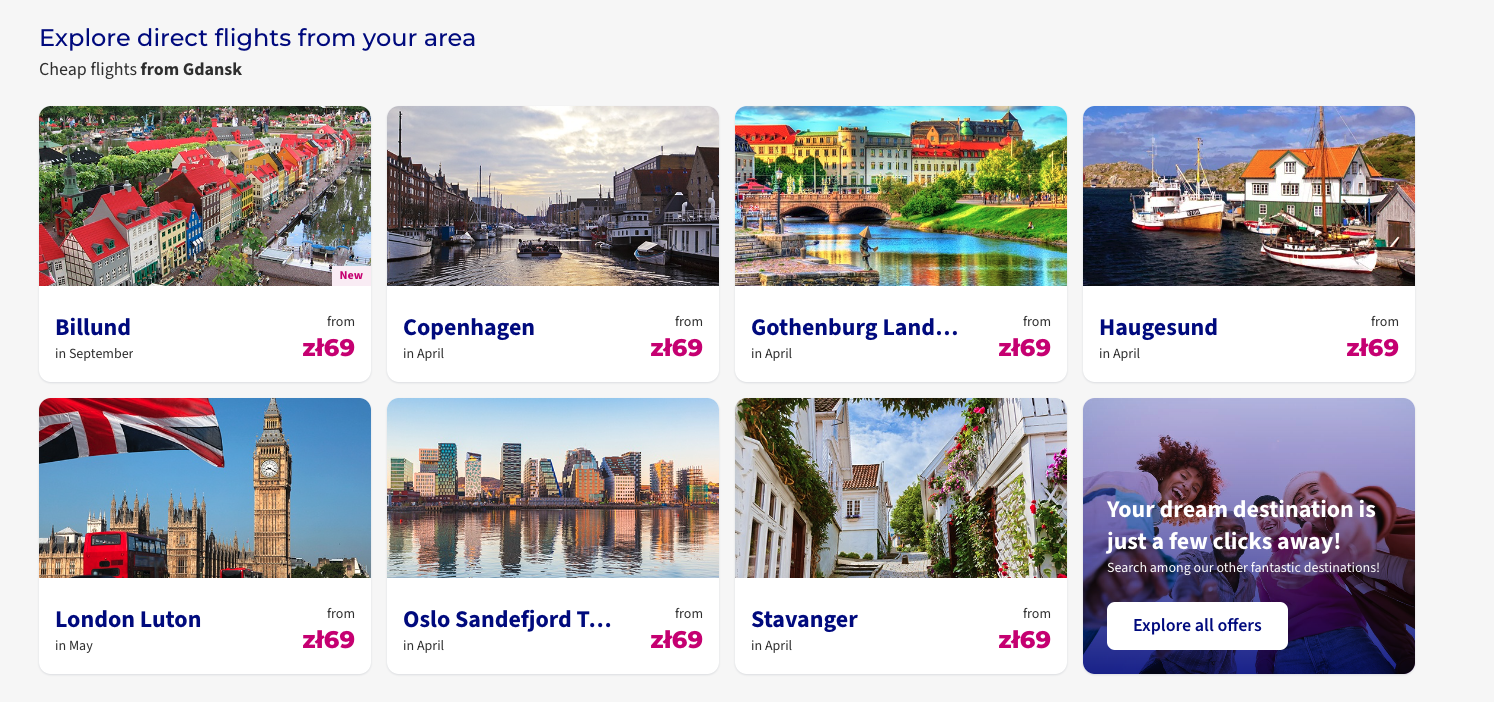
These recommendations are particularly valuable for spontaneous travelers continuously seeking accommodations or attractions. Thanks to geolocation data, the system can immediately suggest the closest available options, saving time when searching through numerous offers.
6. Knowledge-Based Recommendations
Knowledge-based recommendations are a method that utilizes expert knowledge, rules, and information about travel preferences derived from analyzing user data.
This type of recommendation relies on stated or inferred preferences – these might be information provided during registration, answers to profiling questions, or data collected during interactions with the platform. The system takes into account factors such as the preferred vacation type (active, relaxation), the composition of the traveling group (couple, family with children, group of friends), or special requirements (accessibility for people with disabilities, amenities for children).
Additionally, this method employs rules defined by tourism industry experts who create them based on business objectives (considering factors such as promotions, product seasonality, availability, etc.). The expert knowledge created this way can be considered in the recommendations.
7. Content-Based Recommendations
Content-based recommendations are a personalization method that relies on similarities and content analysis on the property’s page.
Techniques used include content similarity (comparing offer descriptions in terms of words and phrases used), topic modeling (automatically detecting main themes in descriptions, e.g., “beach,” “mountains,” “historical sites”), opinion analysis (extracting sentiment and key features from reviews), or clustering (grouping similar offers into sets based on their characteristics).
In tourism, this recommendation is based on detailed features of hotels, restaurants, or attractions (such as atmosphere, standard, type of facility, amenities, location, style, or user ratings).
This solution’s advantage is that it can be implemented without historical data on user behavior. TripAdvisor makes excellent use of this mechanism, displaying a “Similar Experiences” section and often saving bookings that might otherwise be lost due to a lack of availability or a mismatch of the originally selected property.
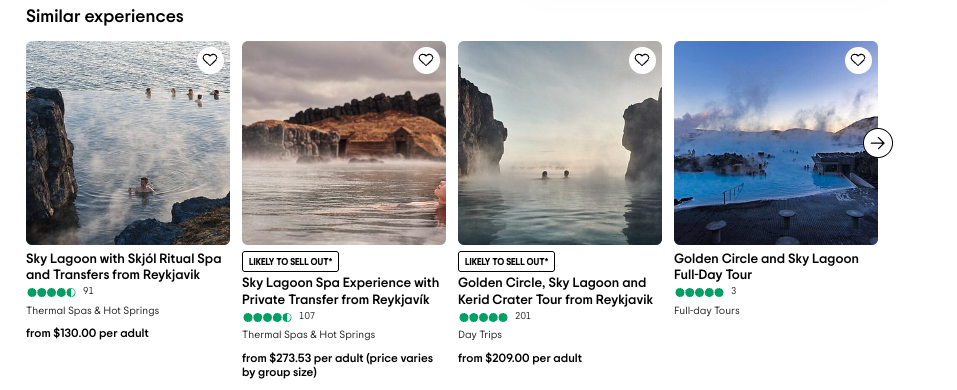
8. Collaborative Filtering Recommendations
Collaborative filtering recommendations are a system that works by recommending items to users that were liked by other users with similar preferences or items similar to those the user has already rated positively.
Example applications of collaborative filtering in the tourism industry include:
- Recommending restaurants that appealed to other travelers with similar culinary preferences
- Suggesting unusual destinations based on the experiences of similar users, which might be missed in typical, general search filters
Collaborative filtering works incredibly well for discovering less obvious, niche places and tourism services liked by travelers that users might not find on their own.
9. Context-Aware Recommendations
Context-aware recommendations are a technique that goes beyond analyzing user preferences and considers the current situation and circumstances in which the user interacts with the system. In the tourism industry, context includes a range of variables that can significantly impact the relevance of recommendations, such as:
- The traveler’s current location
- Season and weather conditions
- Time of day and day of the week
- Ongoing local events and holidays
- Transportation availability
- The user’s device (whether it’s a mobile app or a desktop version)
Some examples of applications in the tourism industry include:
- Recommending air-conditioned attractions during heat waves
- Suggesting indoor attractions during bad weather
- Recommending restaurants that are near the user
- Providing information about local events taking place during the stay
Taking context into account significantly increases the accuracy of tourism recommendations and ensures they are matched not only to the user’s general preferences but also to their specific situation.
10. Session-Based Recommendations
Session-based recommendations are a dynamic personalization mechanism that analyzes user behavior only during their current website visit, without considering their previous history or registered preferences. This is particularly valuable when:
- Users browse offers in incognito mode
- They’re planning trips that are completely different from previous ones
- They’re visiting the site for the first time
The system tracks the sequence of user actions in real-time: viewed properties, applied filters, search criteria entered, and time spent on particular offers. Based on this information, it quickly builds a temporary profile of interests and adapts recommendations to current needs.
Examples of applications include:
- Suggesting properties similar to those the user has just viewed
- Recommending hotels that meet the criteria the user has most frequently filtered during the session
- Proposing attractions that match the character of the viewed properties
- Reminding users about offers viewed during the current session
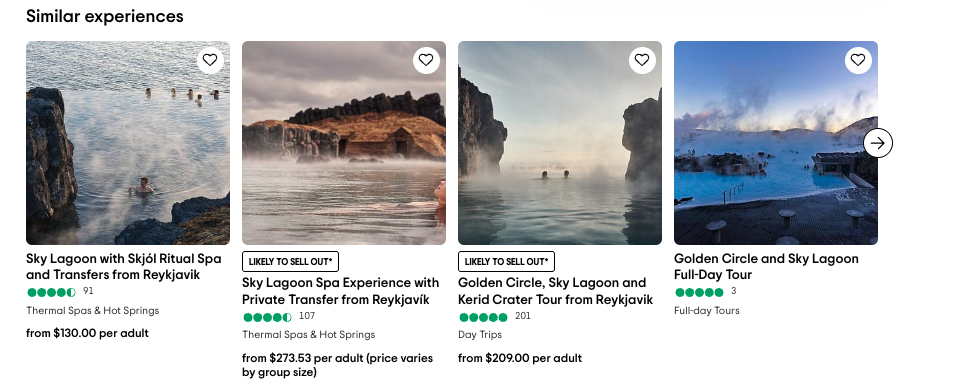
This type of recommendation is particularly effective for platforms with high one-time user traffic and for people whose travel preferences significantly change depending on the purpose of the trip.
Where to Present Tourism Offer Recommendations?
The effectiveness of recommendation systems largely depends on their proper placement on a website or application. Depending on their type and business goals, recommendations should be presented in various locations on a tourism platform.
Optimal recommendation locations based on business goals:
- Increasing click-through rate: homepage, destination category pages, specific property pages, email campaigns, and display ads
- Improving conversion rate: specific tourist property description page
- Maximizing revenue per session: shopping cart, reservation completion page
Types of recommendation models and their optimal placement:
- Recommended for You: Works best on the homepage or user panel, based on the customer’s search history and bookings. They’re excellent as inspiration during the initial stage of travel planning.
- Others You May Like: These are usually displayed on a specific property description page. They show alternative hotels or attractions with similar characteristics. They’re handy when the viewed property doesn’t meet all expectations or is unavailable on the selected dates.
- Frequently Booked Together: This recommendation is most often found in the cart or on the booking page. It suggests complementary services such as transfers, travel insurance, or optional excursions.
How to Implement Recommendation Systems in a Travel Website?
Implementing an effective recommendation system in the tourism industry requires specialized technological solutions. The best approach is to use dedicated recommendation engines that offer advanced personalization capabilities through machine learning mechanisms and learning to rank.
One such solution is the Qtravel Search&Discovery engine, which was created specifically for advanced tourism offer recommendations. This tool enables personalization of user experiences at every stage of travel planning, from inspiration to booking completion.
See How Recommendations Can Transform Your Business
Recommendation systems are a real game-changer in the tourism industry. From simple suggestions of similar hotels, through context-based personalization, to intelligent analysis of user behaviors – all these mechanisms have one goal: to help your company improve sales and customer satisfaction.
Although implementing such technology might seem complicated, you don’t need to reinvent the wheel. Modern recommendation engines, such as Qtravel Search&Discovery, do most of the heavy lifting for you. Check out the Qtravel Search demo and see how intelligent recommendations can transform the way you present offers to your customers.